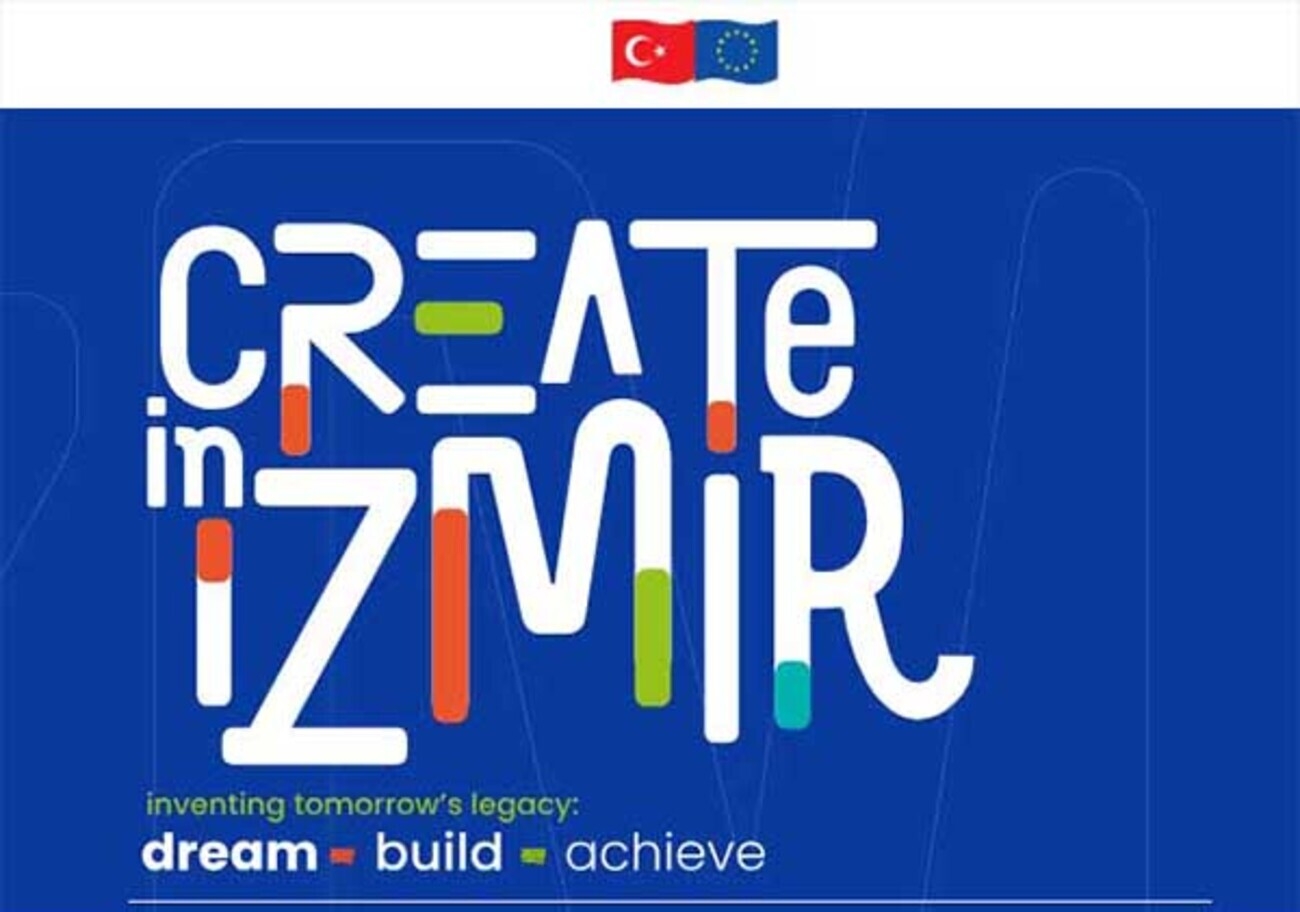
Izmir University of Economics Information Technologies Hackathon Event
Izmir University of Economics IT Hackathon was held on 21-22 December 2024 with the participation of 22 of our students ...
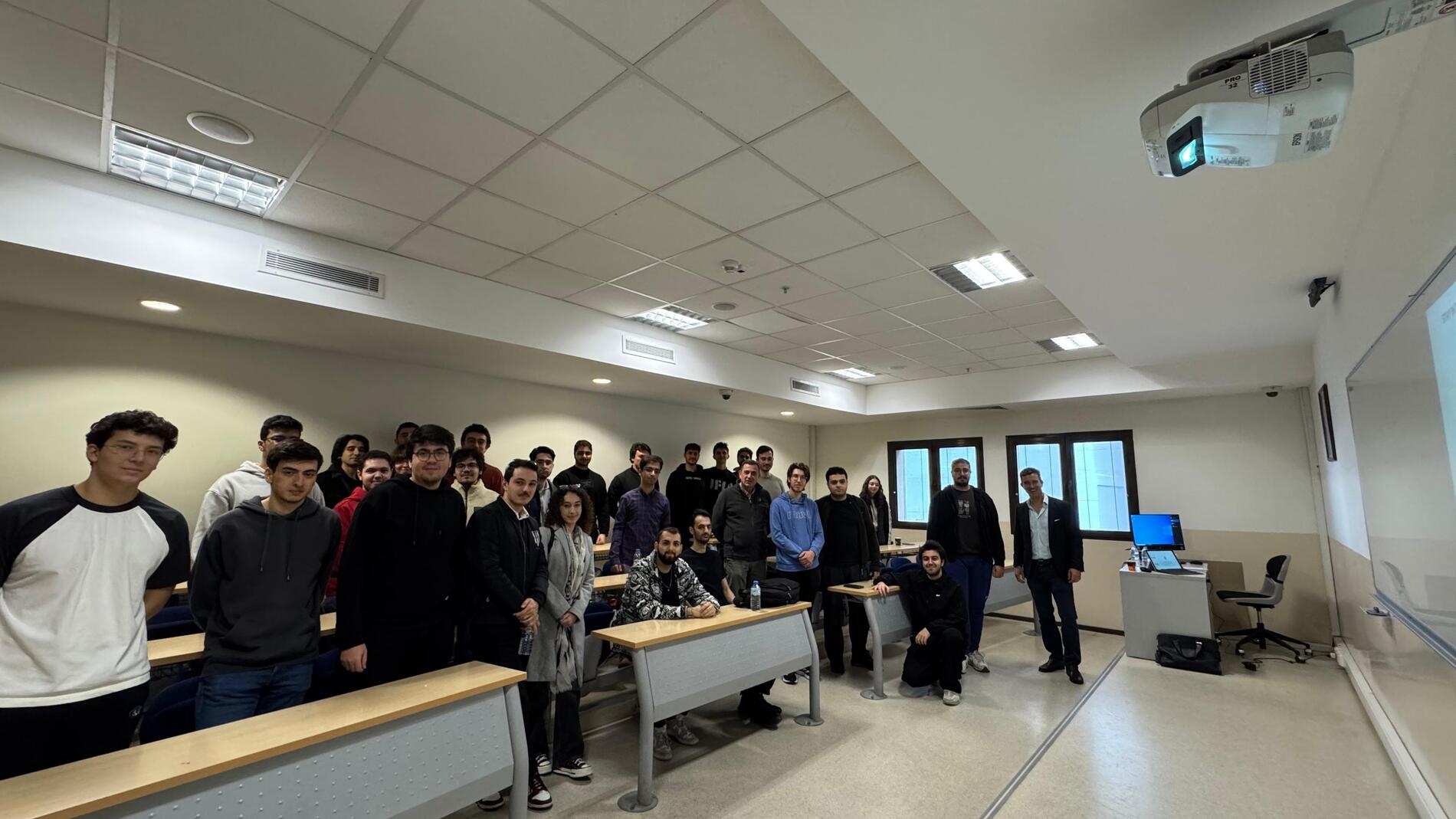
Visit of broadAngle in Izmir University of Economics
Garrison Atkisson, co-founder and CEO of broadAngle, a software company operating in the US and Izmir, and Nihatcan Çolpan, a ...